Comparison of Methods for Parking Availability Prediction
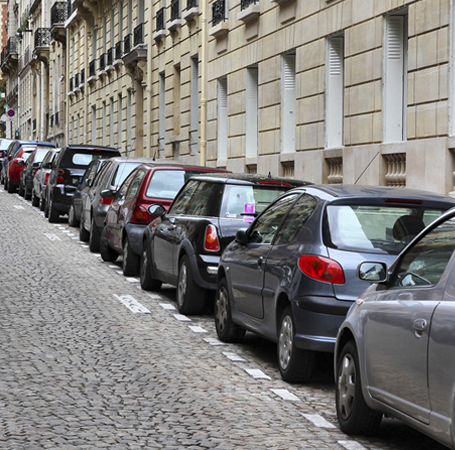
Team: | Supriya Gurupadaswamy |
Jahr: | 2017 |
Laufzeit: | 2017 |
Ist abgeschlossen: | ja |
Parking availability prediction is an interesting domain which needs to be explored in order to make the search of a parking place easier, which could in turn save time and energy. Employing pre-existing data (sequential parking data) to develop a model for future prediction is the intent of this thesis. Such a model indirectly reduces the need of static sensors to collect data every five minutes on a daily basis.Different methods, namely, linear regression, Long Short Term Memory-LSTM (a specific recurrent neural network) and Auto regressive integrated moving average (ARIMA) are used in the modeling of data to predict the parking availability. These methods are compared to suggest a prediction method which is efficient. Different time series components like trend, seasonality and residual components are studied and their behavior on predictions is covered. Also, additional features like weather, type of day, time of day are studied to check whether these features are influential to the prediction and if it improves the prediction.