Reinforcement learning-based sharing data selection for collective perception of connected autonomous vehicles
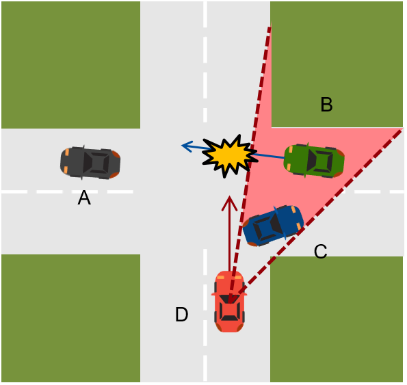
Leitung: | Prof. Markus Fidler, Prof. Monika Sester, Yunshuang Yuan, Shule Li, Sören Schleibaum |
Team: | Yuqiao Bai |
Jahr: | 2021 |
Ist abgeschlossen: | ja |
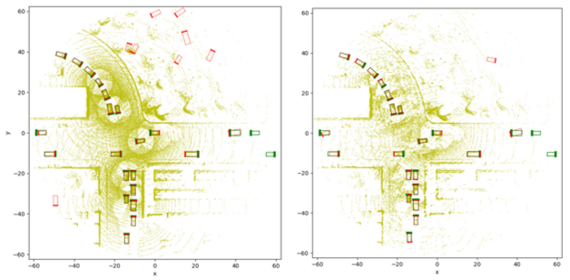
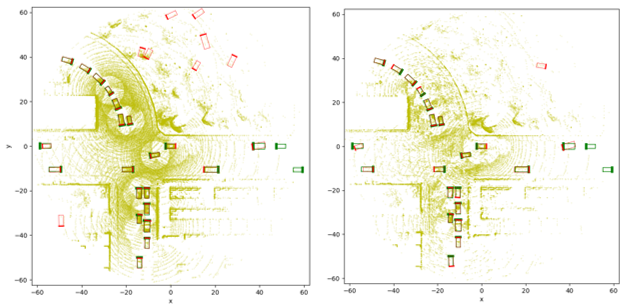
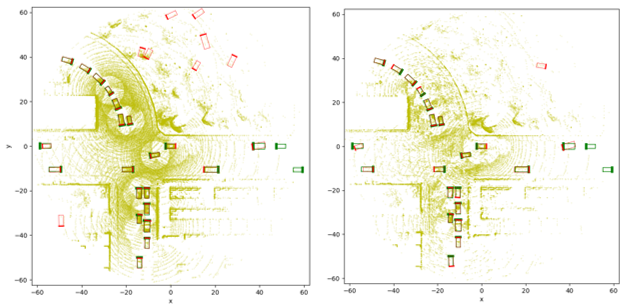
The capability of environmental perception by individual vehicles is very limited. Single-view or single-agent perception, such as 3D target detection, always has unsolvable problems, such as limited viewing angle, low point cloud density, and occlusion (as shown in the first figure). In contrast, multiple spatially sparsely distributed sensors can provide collaborative sensing, which makes it possible to solve these problems. The sensing capabilities of Connected Autonomous Vehicles (CAVs) can be enhanced via collective perception. However, sharing Collective Perception Messages (CPM) in the CAV networks should be efficient enough in the limited communication resources.
In this thesis, a deep reinforcement learning model is proposed to reduce the redundancy of CPMs for the raw point cloud data sharing scenario in the CAV networks. By combining deep reinforcement learning with collaborative perception, a RL based method for selecting collective perception data that uses the DDQN algorithm is implemented. Through this model, the vehicle can intelligently select the data to be transmitted, thereby eliminating redundant data in the network, saving limited network resources, and reducing the risk of communication network congestion.
The result in the second figure (left: before reduction, right: after reduction) shows that through the RL-based CPM data selection method, the amount of data transmitted is greatly without detection performance drop. Moreover, many wrong positive detection are also removed.