Deep Learning for Flood Relevant Images and Texts from Social Media
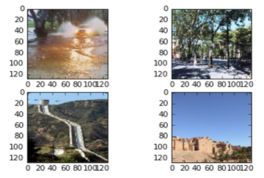
Led by: | Yu Feng, Prof. Brenner |
Team: | Sergiy Shebotnov |
Year: | 2018 |
Is Finished: | yes |
Floods are among Earth's most common and most destructive natural hazards. This work explores the idea of utilizing user-generated information from social media to recognize early signs of flood relevant events. The goal of this work lies in the development and implementation of a Deep Learning solution with the ability to detect the presence of flood relevant events from user-generated images and texts.
Floods are among Earth's most common and most destructive natural hazards. This work explores the idea of utilizing user-generated information from social media to recognize early signs of flood relevant events. The goal of this work lies in the development and implementation of a Deep Learning solution with the ability to detect the presence of flood relevant events from user-generated images and texts. For this task, convolutional neural network and recurrent neural network models are combined into single multimodal neural network model to be used for classification. Both single models make use of transfer learning to improve performance. For images, pre-trained convolutional neural network is reused for this task. For text, pre-trained word embeddings are used to help with text classification. Additionally, hyperparameter tuning is performed on the RNN model to find optimal values of model parameters. All models are evaluated on the MediaEval 2017 and 2018 dataset and compared with other participating groups or individuals. For the most part, all proposed models show competitive or adequate results. Overall it can be concluded, that using visual and textual information from social media to identify flood relevant events is possible with a relatively high degree of accuracy to make it useful for emergency monitoring.