The Institute of Cartography and Geoinformatics is an institute of the Faculty of Civil Engineering and Geodetic Science. We do research and teaching in the field of automatic analysis of geospatial data - with applications in automatic mapping, robotics and autonomous driving.
Examples of our Research and Teaching
News
Paper published in PFG: Conditional Adversarial Networks for Multimodal Photo-Realistic Point Cloud Rendering
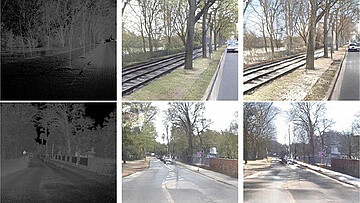
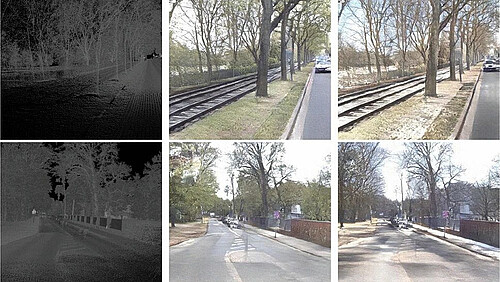
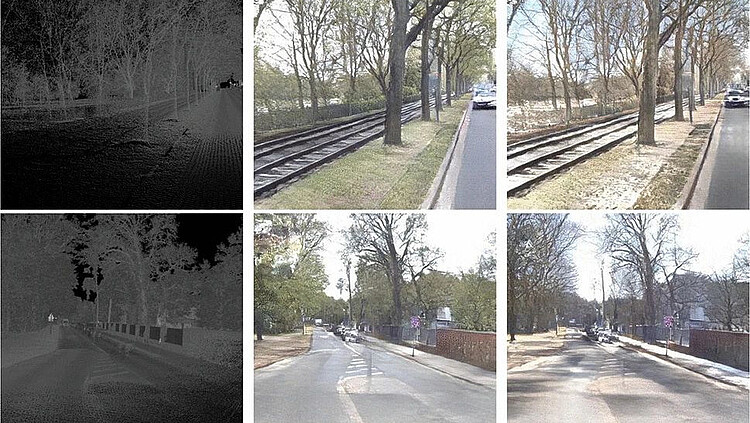
Torben Peters and Claus Brenner developed a method to create photorealistic visualizations from point clouds.
We investigate whether conditional generative adversarial networks (C-GANs) are suitable for point cloud rendering. For this purpose, we created a dataset containing approximately 150,000 renderings of point cloud–image pairs. The dataset was recorded using our mobile mapping system, with capture dates that spread across 1 year. Our model learns how to predict realistically looking images from just point cloud data. We show that we can use this approach to colourize point clouds without the usage of any camera images. Additionally, we show that by parameterizing the recording date, we are even able to predict realistically looking views for different seasons, from identical input point clouds.
Events
Paper published in PFG: Conditional Adversarial Networks for Multimodal Photo-Realistic Point Cloud Rendering
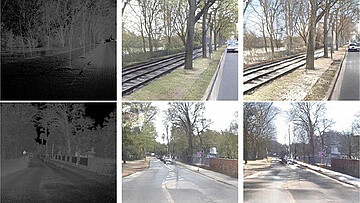
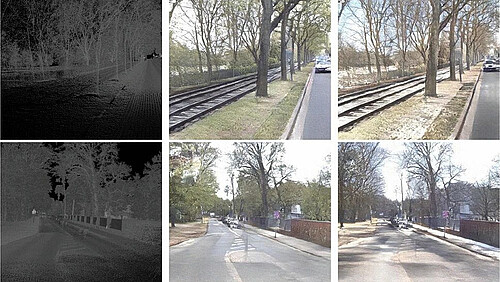
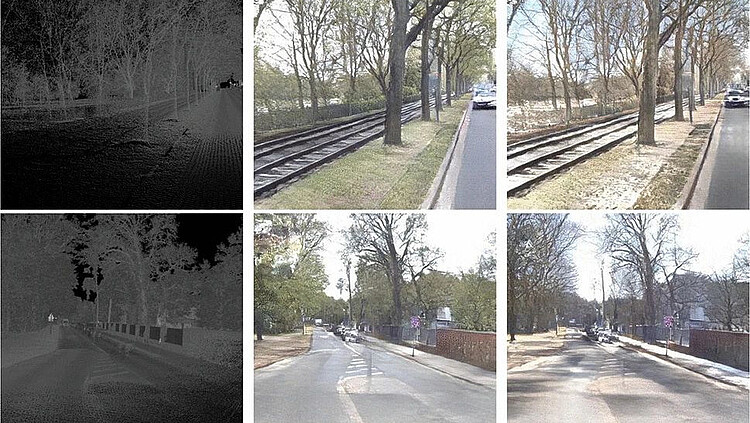
Torben Peters and Claus Brenner developed a method to create photorealistic visualizations from point clouds.
We investigate whether conditional generative adversarial networks (C-GANs) are suitable for point cloud rendering. For this purpose, we created a dataset containing approximately 150,000 renderings of point cloud–image pairs. The dataset was recorded using our mobile mapping system, with capture dates that spread across 1 year. Our model learns how to predict realistically looking images from just point cloud data. We show that we can use this approach to colourize point clouds without the usage of any camera images. Additionally, we show that by parameterizing the recording date, we are even able to predict realistically looking views for different seasons, from identical input point clouds.